QBUS3820 Lecture Notes - Lecture 3: Model Selection, Test Statistic, Mahalanobis Distance
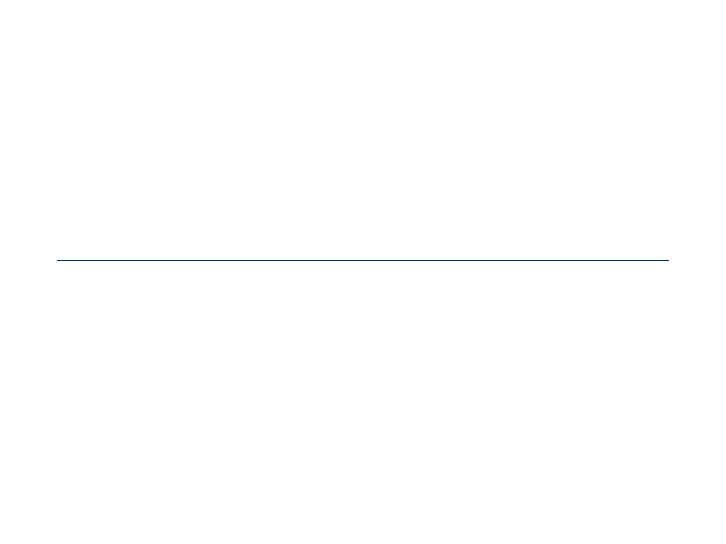
QBUS3820: Machine Learning and Data
Mining in Business
Lecture 3: Linear Regression and K-Nearest Neighbours
Associate Prof. Peter Radchenko
Semester 1, 2018
Discipline of Business Analytics, The University of Sydney Business School
find more resources at oneclass.com
find more resources at oneclass.com
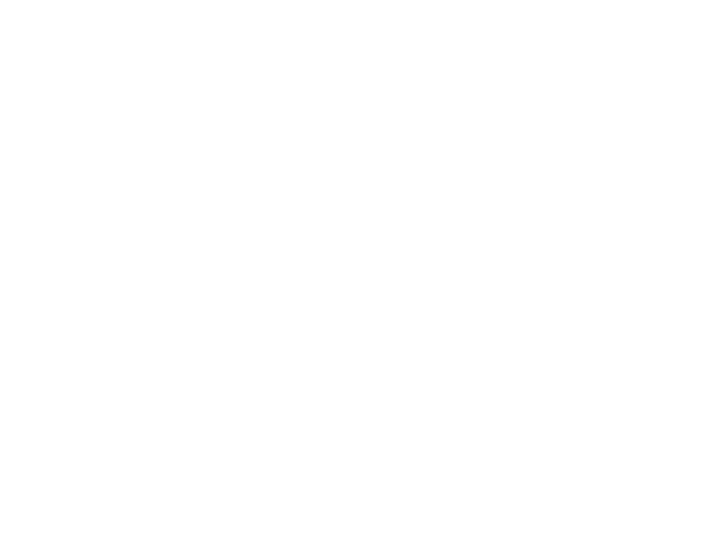
Lecture 3: Linear Regression and K-Nearest Neighbours
1. Statistical properties of OLS
2. The Gaussian MLR model
3. K-Nearest Neighbours
4. Comparison with linear regression
2/40
find more resources at oneclass.com
find more resources at oneclass.com
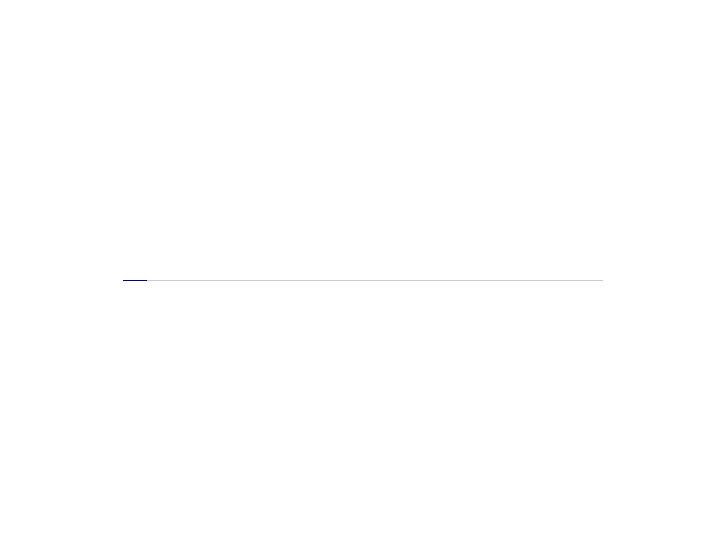
Statistical properties of OLS
find more resources at oneclass.com
find more resources at oneclass.com
Document Summary
Discipline of business analytics, the university of sydney business school. Lecture 3: linear regression and k-nearest neighbours: statistical properties of ols, the gaussian mlr model, k-nearest neighbours, comparison with linear regression. In classical statistics, the population parameter is xed and the data is a random sample from the population. We estimate by applying an estimator b to data (in our case the ols estimator). As the sample on which estimator b is computed is random, the value of the estimator is a random variable, and the distribution of this random variable is called the sampling distribution. We can study the uncertainty of an estimate by understanding the sampling distribution of the estimator. Suppose that we draw a large number of di erent datasets d(s) (s = 1, . On each of these datasets, we apply the estimator b and obtain a set of estimates {b (d(s))}s thought of as the distribution of these estimates as we let s . s=1.