PUBH2007 Lecture Notes - Lecture 2: Pie Chart, Confidence Interval, Confounding
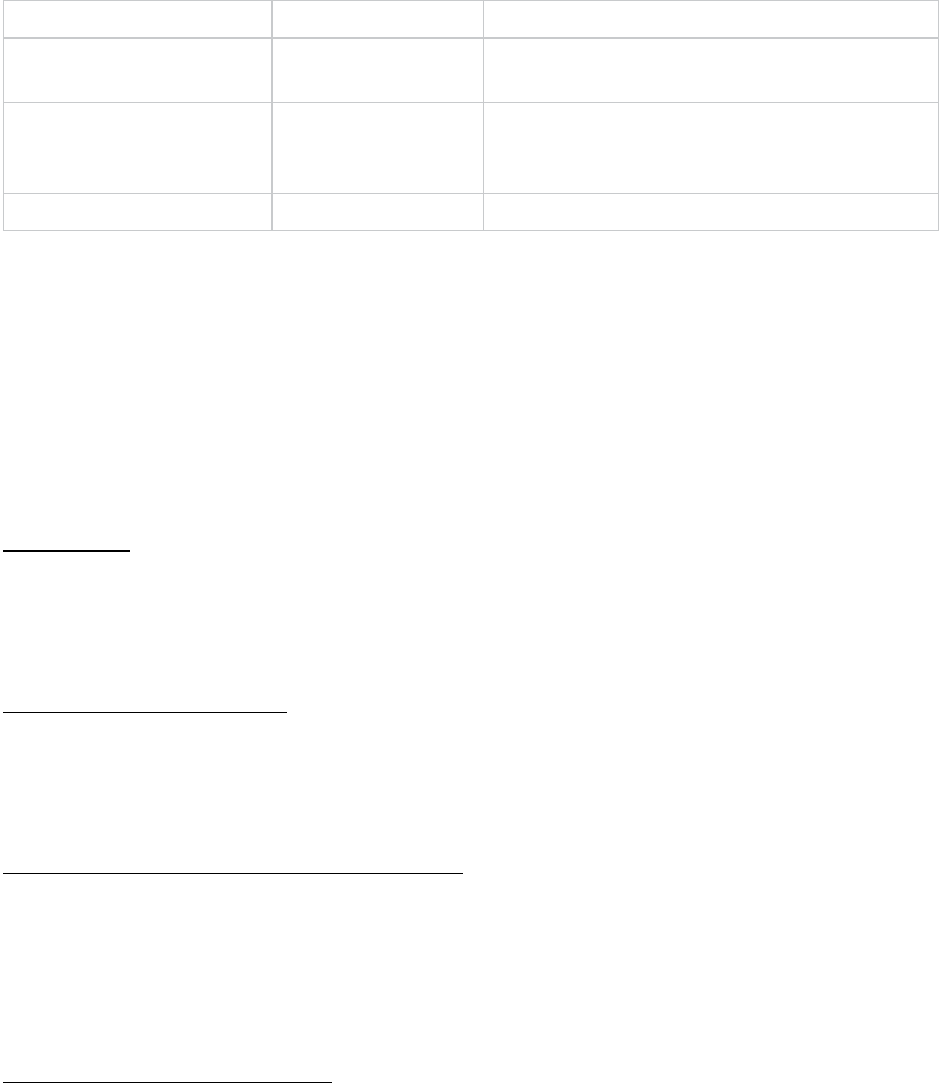
PUBH2007 LECTURE TWO
Two variables
Presentation of data
Summarise relationship
Both quantitative (numeric)
Scatter plot
Correlation & regression
(this week & week 11)
One categorical, one
numeric
Side by side box-plots
(one for each
category)
Comparisons of means and other statistics (week
7)
Both categorical
Two-way table
Chi-squared test (week 9)
Cases = rows (i.e. ID number)
Data = columns
E.g. correlation and regression data illustrated by an example about weight (kg), height, and waist
circumference.
We want to know which variables are most closely related to weight and how well you could predict the
weight of a young male if you knew his height
SCATTERPLOT
• The most useful graph for displaying the relationship between two quantitative variables
• Shows the relationship between two quantitative variables measured on the same individuals
• One variable on x axis, one variable on y axis
• Each individual in the data appears as a point on the graph
HOW TO MAKE A SCATTERPLOT
1. Decide which variable should go on each axis. If a distinction exists, plot the explanatory variable on
the x-axis and the response variable on the y-axis
2. Label and scale your axes
3. Plot individual data values
R INSTRUCTIONS FOR PRODUCING A SCATTERPLOT
Graphs > scatterplot
HOW TO INTERPRET A SCATTERPLOT
As in any graph of data, look for the overall pattern and for striking departures from that pattern.
Described as DIRECTION, FORM AND STRENGTH
find more resources at oneclass.com
find more resources at oneclass.com
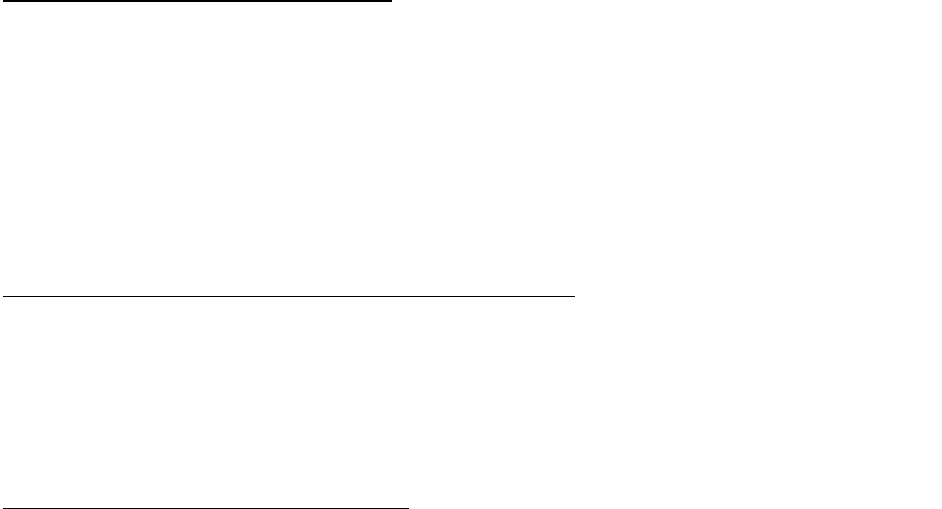
• 'Direction' = Positive relationship i.e. mass increases with height
i.e. do variables increase together or in opposite directions?
• 'Form' = linear/curved
• 'Strength' = how much the data is scattered about
Explanatory = x axis
Response = y axis
E.g. standing height of one individual vs mass of the same individual
Correlation coefficient: denoted by r (or R)
r is a single number (statistic) which summarises strength of a straight line relationship between two
variables
Don’t need to know formula - need only to interpret
Always plot data so you can see the patterns and know whether a straight line is a sensible summary of
the relationship
R INSTRUCTIONS FOR OBTAINING A CORRELATION COEFFICIENT
Statistics > summaries > correlation test
**Need to hold CTRL key when picking second variable in a correlation calculation
R OUTPUT FOR A CORRELATION COEFFICIENT
> cor.test(anthrop_male$Mass, anthrop_male$Standing, alternative="two.sided",
+ method="pearson")
Pearson's product-moment correlation: where Pearson's r is the usual summary statistic to use
data: anthrop_male$Mass and anthrop_male$Standing
Below: Will come up later in course: tells us it is extremely unlikely (probability 7×10-5) that the
correlation is zero; in fat, we’re 9% sure it is etween 0. and 0.7
t = 4.401, df = 42, p-value = 7.247e-05
alternative hypothesis: true correlation is not equal to 0
find more resources at oneclass.com
find more resources at oneclass.com
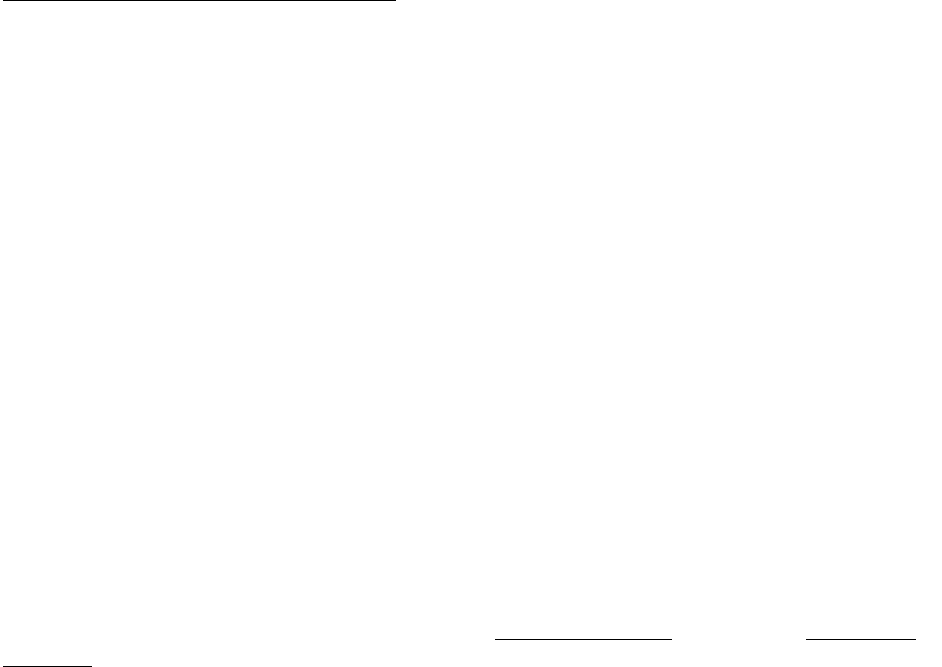
95 percent confidence interval:
0.3179427 0.7359320
Correlation r = 0.56
sample estimates:
cor
0.5617947
PROPERTIES OF CORRELATION COEFFICIENT
• R is always between -1 and +1
• R = -1 if data are in a (perfect) straight line and the relationship is negative (i.e. as one variable
increases the other decreases)
• R = +1 if data are in a (perfect) straight line and the relationship is positive (i.e. they increase or
decrease together)
• R = 0 if there is no straight line relationship (but there could be some other relationship, e.g.
curved)
• If a negative r value <0.5 or a positive r value >0.5, this suggests there is a substantial straight line
relationship between the variables
• For normally distributed data, use PEARSON'S CORRELATION COEFFICIENT
• If data are not approximately Normally distributed, use SPEARMAN'S CORRELATION COEFFICIENT
Formula:
REGRESSION provides an alternative tool when it makes sense to think of one variable being an
OUTCOME or RESPONSE and the other being an EXPLANATOR or PREDICTOR variable (i.e. age)
A regression line is a straight line that describes how a response variable y changes as an explanatory
variable x changes.
We can use a regression line to predict the value of y for a given value of x
A simple linear regression model is an equation showing the mathematical relationship between
response variable, y and one explanatory variable, x and the variation of points about the line
E.G. equation for the relationship between y (e.g. mass) and x (e.g. standing height) is:
^Y = a +bx
X = the value of the explanatory variable
find more resources at oneclass.com
find more resources at oneclass.com
Document Summary
Correlation & regression (this week & week 11) Side by side box-plots (one for each category) E. g. correlation and regression data illustrated by an example about weight (kg), height, and waist circumference. We want to know which variables are most closely related to weight and how well you could predict the weight of a young male if you knew his height. Scatterplot: the most useful graph for displaying the relationship between two quantitative variables, one variable on x axis, one variable on y axis, each individual in the data appears as a point on the graph. How to make a scatterplot: decide which variable should go on each axis. If a distinction exists, plot the explanatory variable on. Shows the relationship between two quantitative variables measured on the same individuals the x-axis and the response variable on the y-axis: label and scale your axes, plot individual data values.