PHIL2420 Lecture Notes - Lecture 2: Null Hypothesis, Spontaneous Remission, Gastroenteritis
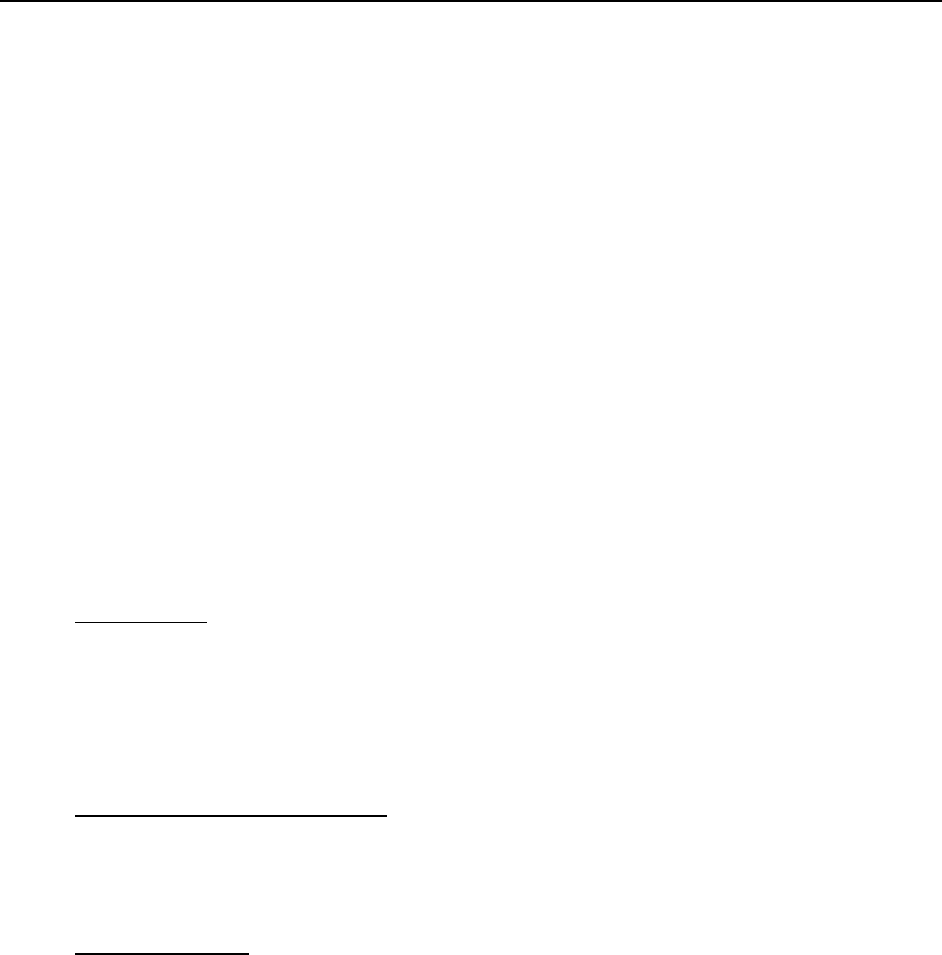
PHIL 2420
Critical Thinking
March 8, 2018
WEEK 2
Reasoning Causally
Correlation
• Usually we think we’re observing causes
• We automatically see causes
• If we think critically, we only ever observe correlations
• Experiments usually talk about correlations
o Likelihood of correlation between variables
o Can infer something about causality
• Data are correlated when associated in an empirical investigation
• EXAMPLE: Red sky and sun coming up, redness is always associated with the
sunrise
• Can we move beyond mere correlation?
Correlation Causality – statistically related doesn’t mean there’s a causal relation
between the two
• Don’t know the direction of the causal relationship
o Is red sky before the sunrise?
o Is it because this was observed first?
1. Coincidence
a. EXAMPLE: In past 10 years, there has been an increase in reality TV
shows and number of women who attend university
b. Might be related, but not exactly
c. Can happen purely by accident
d. EXAMPLE: Dream about someone shooting. Mass shooting happens the
next day in America. No causal relationship to these things.
2. Reversal of Cause and Effect / Reversed Causality
a. EXAMPLE: Algal bloom was correlated with a fish kill. Scientists conclude
algal bloom caused the fish kill. Later studies showed algae grew where
fish died
b. One might cause the other
3. Common Cause
a. EXAMPLE: Possible correlation between myopia and children sleeping
with light on. Later, myopic parents were seen to more likely leave the
lights on in their children’s room
b. There’s a causal relationship
c. Cause was genetics
From Correlation to Causality – David Hume, 1739
1. Data are always correlated
find more resources at oneclass.com
find more resources at oneclass.com
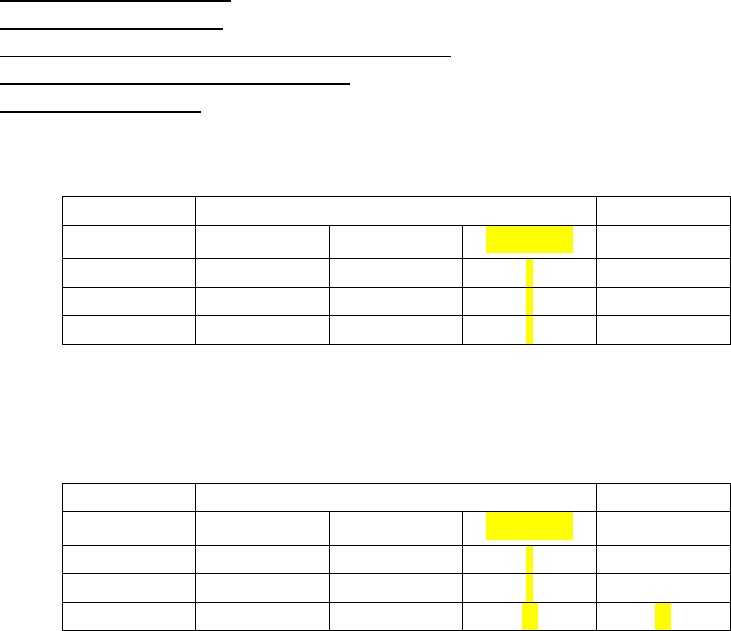
o Not always
2. Cause preceded the effect
3. The effect is the result of the cause
Causal relationships must be inferred
• Cannot directly observe causes
• You think you see the causes of things all the time
• EXAMPLE
o Car not working
o Putting petrol but it’s not working
• We usually don’t see the actual causes
Mill’s Methods – not perfect, but useful
1. Identify a set of candidate causes - Events or conditions that happened before E,
and one of them is supposed to be the cause of E
2. Collect information about range of situations involving these candidate causes
and determine whether E occurred afterward in these situations
3. Based on the information collected, use one of the 5 rules below to infer the
cause of E
Methods
1. Method of Agreement
2. Method of Difference
3. Joint Method of Agreement and Difference
4. Method of Concomitant Variation
5. Method of Residue
EXAMPLE: Going to a restaurant with friends and some get gastroenteritis (E)
1. Agreement
Situation
Candidate Cause
Effect
Albacore
Beef
Chicken
Sick
Paige
/
X
/
/
Quinn
X
/
/
/
Roger
/
/
/
/
a. If two or more situations leading to an effect E has only one event C in
common, then C is the cause of E
2. Difference
Situation
Candidate Cause
Effect
Albacore
Beef
Chicken
Sick
Paige
/
/
/
/
Quinn
/
/
/
/
Roger
/
/
X
X
find more resources at oneclass.com
find more resources at oneclass.com
Document Summary
Correlation: usually we think we"re observing causes, we automatically see causes, experiments usually talk about correlations. Correlation causality statistically related doesn"t mean there"s a causal relation between the two: don"t know the direction of the causal relationship. Mass shooting happens the next day in america. No causal relationship to these things: reversal of cause and effect / reversed causality, example: algal bloom was correlated with a fish kill. Scientists conclude algal bloom caused the fish kill. Later studies showed algae grew where fish died: one might cause the other, common cause, example: possible correlation between myopia and children sleeping with light on. Later, myopic parents were seen to more likely leave the lights on in their children"s room: there"s a causal relationship, cause was genetics. From correlation to causality david hume, 1739: data are always correlated, not always, cause preceded the effect, the effect is the result of the cause.