ECON1203 Lecture Notes - Lecture 8: Central Limit Theorem, Statistical Hypothesis Testing, Standardized Test
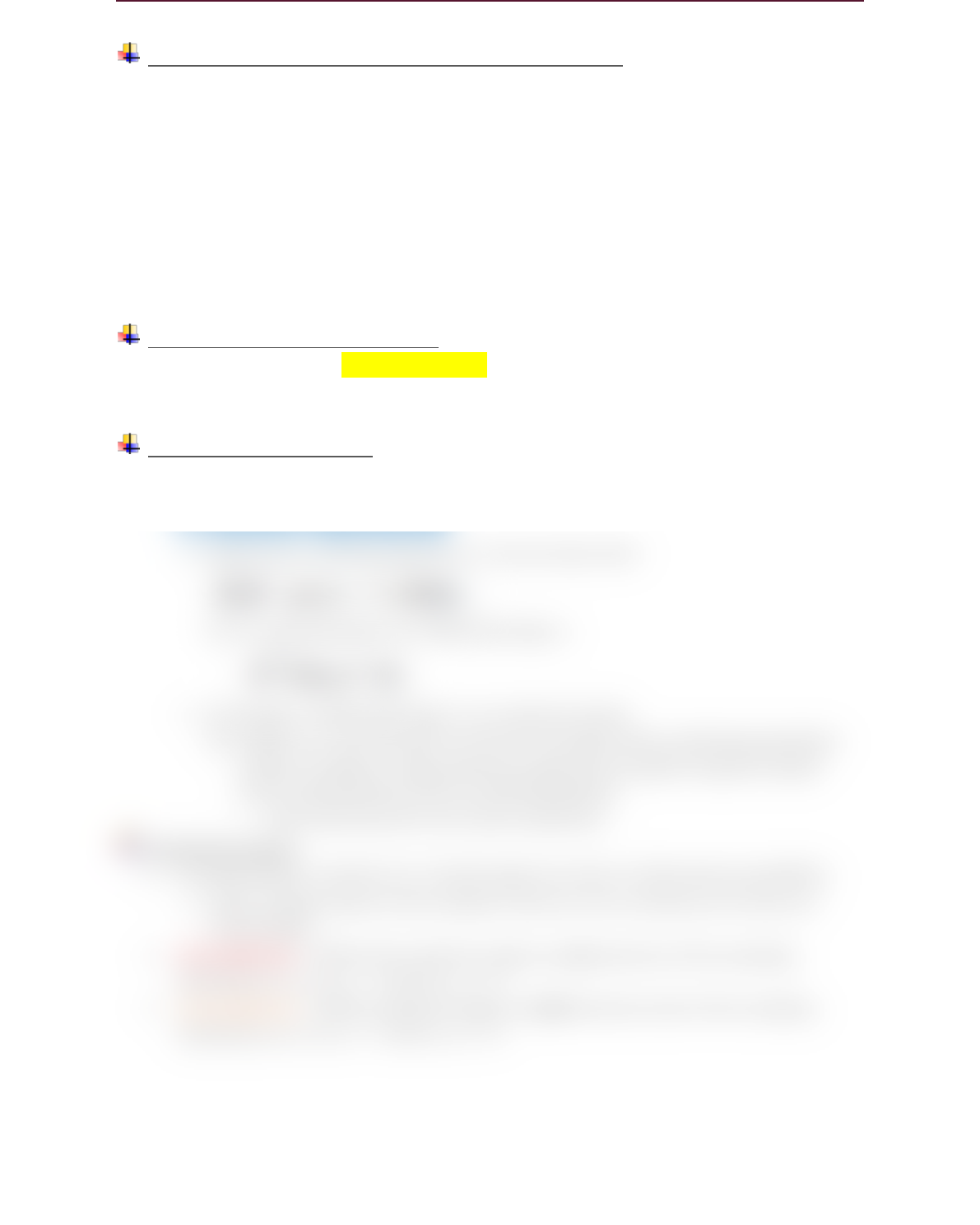
7 – Central Limit Theorem, Hypothesis Testing cont.
Properties of the sampling distribution of the sample mean
• E (X bar) = µ
• Var (X bar) = 2 / n
sd (X bar) = / n
• General expressions for the mean and variance of the sampling distribution of the
sample mean
• May not be enough to characterise the whole sampling distribution
• Exact sampling distribution is known in 2 cases:
The population distribution of X is normal
The population distribution of X is not normal but n is large
Sampling from a normal distribution
• If X ~ N (µ, 2), then X bar ~ N (µ, 2/n)
The sample mean is also normal, just like the population random variable, as it is
a liner combination of normal random variables
Central Limit Theorem (CLT)
• The sampling distribution of the mean of a random sample drawn from any
population with mean µ and variance
2 will be approximately normally distributed
for a sufficiently large sample size
Based upon a limiting argument, it can be shown that:
(a) Or, approximately, for sufficiently large n,
How large is suffiietly large is a empirical matter
(a) Implies it is not necessary to assume normality of the underlying population
random variable to make inferences about this variable using the sample
mean and properties of this normal distribution
i. Extremely powerful and useful implication
Hypothesis testing
• Formally define a rejection (or critical) region by choice of alternative possibilities
Other possible values of test statistic, that are not so extreme, lie in the non-
critical region
• One-tailed test – defines the rejection region as one extreme of the sampling
distribution (i.e. H0: µ = .7 and H1: µ > .7)
• Two-tailed test – defines rejection region as both extreme ends of the sampling
distribution (i.e. H0: µ = .7 and H1: µ ≠ .7)
find more resources at oneclass.com
find more resources at oneclass.com
Document Summary
7 central limit theorem, hypothesis testing cont. Properties of the sampling distribution of the sample mean: e (x bar) = , var (x bar) = 2 / n. The population distribution of x is normal. The population distribution of x is not normal but n is large. If x ~ n ( , 2), then x bar ~ n ( , 2/n) The sample mean is also normal, just like the population random variable, as it is a liner combination of normal random variables. Central limit theorem (clt: the sampling distribution of the mean of a random sample drawn from any population with mean and variance 2 will be approximately normally distributed for a sufficiently large sample size. Based upon a limiting argument, it can be shown that: (a) or, approximately, for sufficiently large n, Hypothesis testing: formally define a rejection (or critical) region by choice of alternative possibilities. Probability of our statistic falling into the rejection region.