PUBH2007 Lecture Notes - Lecture 8: Null Hypothesis, Simple Random Sample, Test Statistic
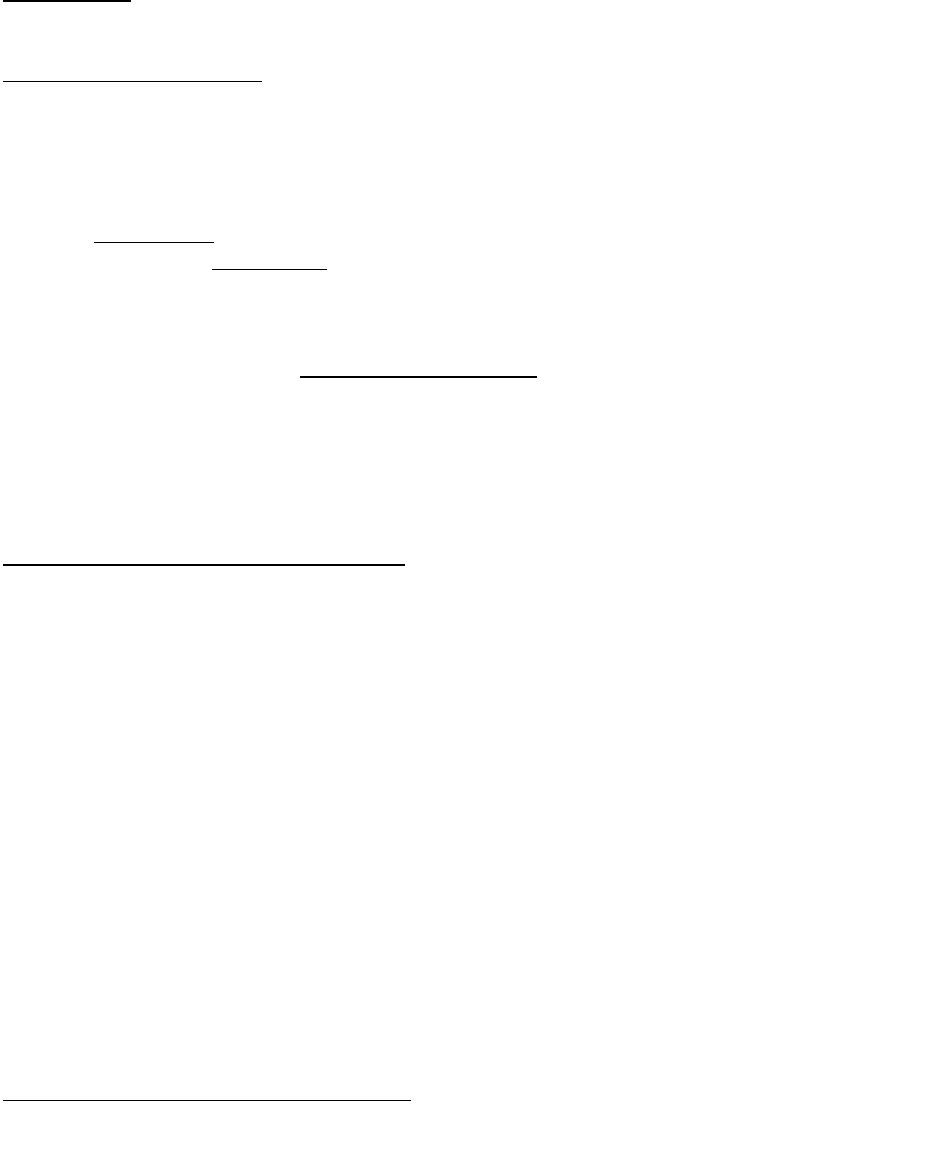
PUBH2007 LECTURE EIGHT
THIS LECTURE:
Proportions
Sampling distribution of one sample proportion
Confidence intervals and significance tests for one proportion
Comparing two proportions
Confidence intervals and significance tests
Lecture 8 and lecture 9 are all about CATEGORICAL VARIABLES
RECALL: Binomial setting
1. There is a fixed number n of observations (e.g., number of individuals in a sample.)
2. The n observations are independent, i.e., knowing the result of one observation does not change the
probabilities of other observations
3. Each observation falls into one of just two categories, sometimes called suess or failure (e.g., girl
or boy)
4. Proaility of suess, p, is the same for each observation
The uer of suesses X from a binomial setting has the binomial distribution with
parameters n and p
n = number of observations
p = proaility of suess at eah oservatio
X has possile values of , , … , n
Inference about the population parameter, p
The population parameter is the probability or proportion of successes in the population, p
It is estimated by the proportion of successes observed in the sample X/n
This is denoted by
pˆ i.e. pˆ=Xn
X-BAR/P-HAT = ESTIMATE
Estimates of p calculated from difference samples will vary, due to sampling variation
This variation is summarised by the standard of
pˆ
SE(pˆ)= p(1−p)n−−−−−−−√
If n is large, the sampling distribution of the
pˆ′s
is approximately Normal with a mean which is the population proportion p (CENTRAL LIMIT THEOREM)
Sampling distribution of a Sample Proportion:
find more resources at oneclass.com
find more resources at oneclass.com
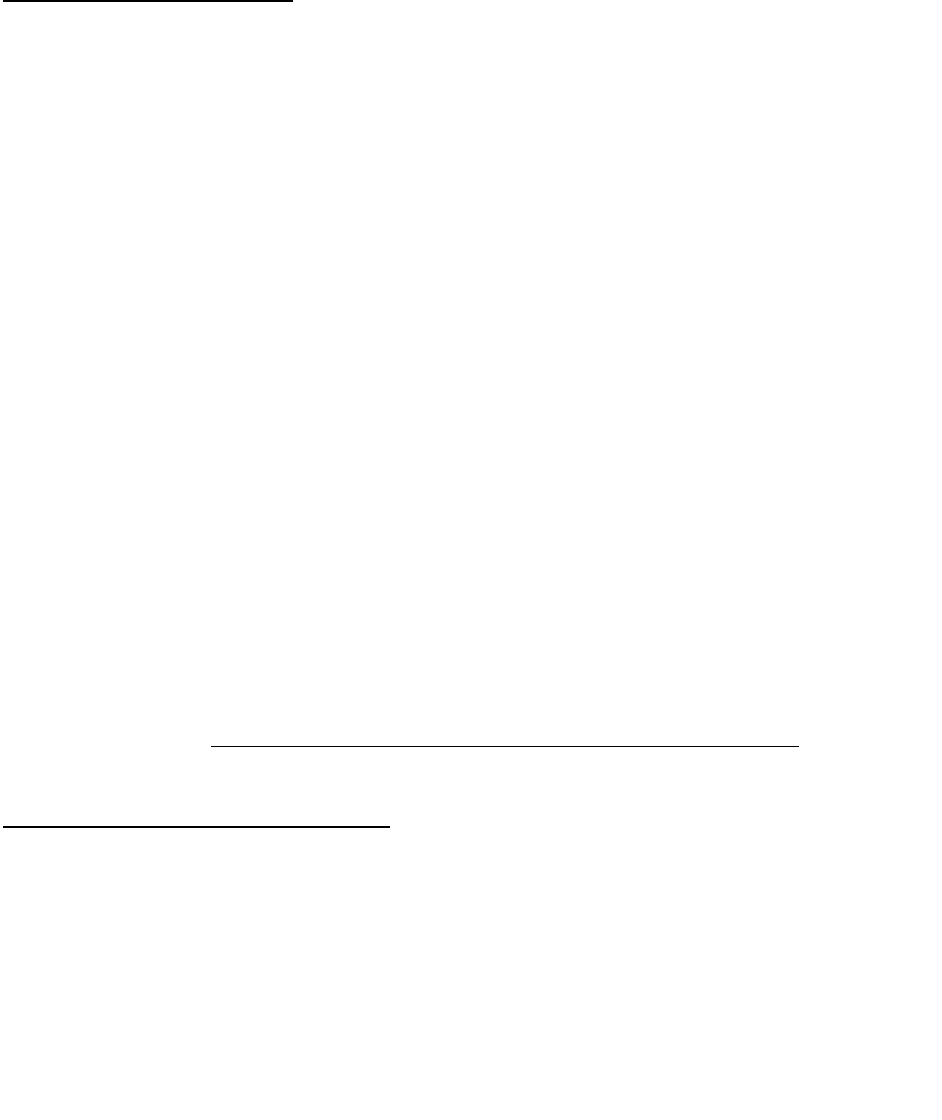
Large Sample CI for a Proportion
We can use the same reasoning as we did with means to construct a CI for an unknown population
proportion p:
pˆ
± margin of error
i.e.
pˆ
± z* standard error
Estimate the SE of the sample proportion by:
Z* FROM RCMDR USING DISTRIBUTIONS > QUANTILES
NOTE: We use a z value to calculate a CI for a proportion. We do not use t values because we are not
estimating any extra parameter such as the population SD.
1. Choose a SRS of size n from a large population that contains an unknown proportion p of successes
2. Choose a confidence level. Call it C; e.g. C=95%
3. Then, an approximate level C confidence interval for p is:
Where z* is the value for the standard Normal density curve such that the area between -z* and z* is C.
Use this interval only when the numbers of successes and failures in the sample are large. Roughly, both
should be at least 15 according to MNF.
Calculating Sample Size for one Proportion:
When planning a sample survey, a sample size needs to be decided. It should be based on the desired
margin of error (often based on clinical significance: but don't want sample size to be too large because
expensive).
For a given margin of error, m, a minimum sample size can be calculated.
Margin of error is:
Making n the subject of the equation,
To calculate n we need to know p. But we do not know p!
Obtain a value for p from a pilot study , past experience, or assume p=0.5 if you do not have a good
estimate.
find more resources at oneclass.com
find more resources at oneclass.com
Document Summary
Confidence intervals and significance tests for one proportion. Lecture 8 and lecture 9 are all about categorical variables. The (cid:374)u(cid:373)(cid:271)er of (cid:862)su(cid:272)(cid:272)esses(cid:863) x from a binomial setting has the binomial distribution with parameters n and p n = number of observations p = pro(cid:271)a(cid:271)ility of (cid:862)su(cid:272)(cid:272)ess(cid:863) at ea(cid:272)h o(cid:271)servatio(cid:374) X has possi(cid:271)le values of (cid:1004), (cid:1005), , n. The population parameter is the probability or proportion of successes in the population, p. It is estimated by the proportion of successes observed in the sample x/n. Estimates of p calculated from difference samples will vary, due to sampling variation. This variation is summarised by the standard of p . If n is large, the sampling distribution of the p s is approximately normal with a mean which is the population proportion p (central limit theorem) We can use the same reasoning as we did with means to construct a ci for an unknown population proportion p: p .