ECO220Y5 Lecture Notes - Lecture 9: Random Effects Model, Execution Unit, Null Hypothesis
Multivariate Analysis. Dealing with more than one variable.
In all we have done so far we have only studied the statistical analysis of one variable. The
last part of the course is going to deal with the case where we have to consider more than one
variable. Sometimes the additional variables will be random, sometimes they will not, but in all
cases we will be concerned with the nature of the relationships between the variables. Mostly we
shall concentrate on just two variables and generalize to the case of many. First we shall consider
how to detect whether or not two variables are independent.
A Goodness of Fit Test of Independence.
Imagine two characteristics that an individual may have, say hair colour and eye colour, we
may think of them as two variables and it is of interest to establish whether or not the variables
are distributed independently across individuals. Suppose the entire range of hair colour is
divided into c mutually exclusive and exhaustive categories numbered j = 1,..,c and similarly the
entire range of eye colour is divided into r mutually exclusive and exhaustive categories
numbered i = 1,..,r. The true probabilities that a randomly selected person has a particular hair
colour / eye colour combination can be arranged on an r x c grid whose rows correspond to the
eye colour categories and whose columns correspond to the hair colour categories as in Table 1
below.
The pij’s are joint probabilities of having a particular hair / eye colour combination and taken
together they correspond to the joint probability distribution of the eye colour-hair colour
combinations. The row sums of probabilities (pi.’s) represent the marginal probabilities of having
the i’th eye colouring regardless of which hair colour an individual has and taken together they
constitute the marginal probability distribution of eye colouring. Similarly the column sums
(p.j’s) are marginal probabilities of having the j’th hair colouring regardless of which eye colour
an individual has and taken together they constitute the marginal probability distribution of hair
colouring. Hence all of the pij’s sum to one as does the sum of the pi.’s and the sum of the p.j’s.
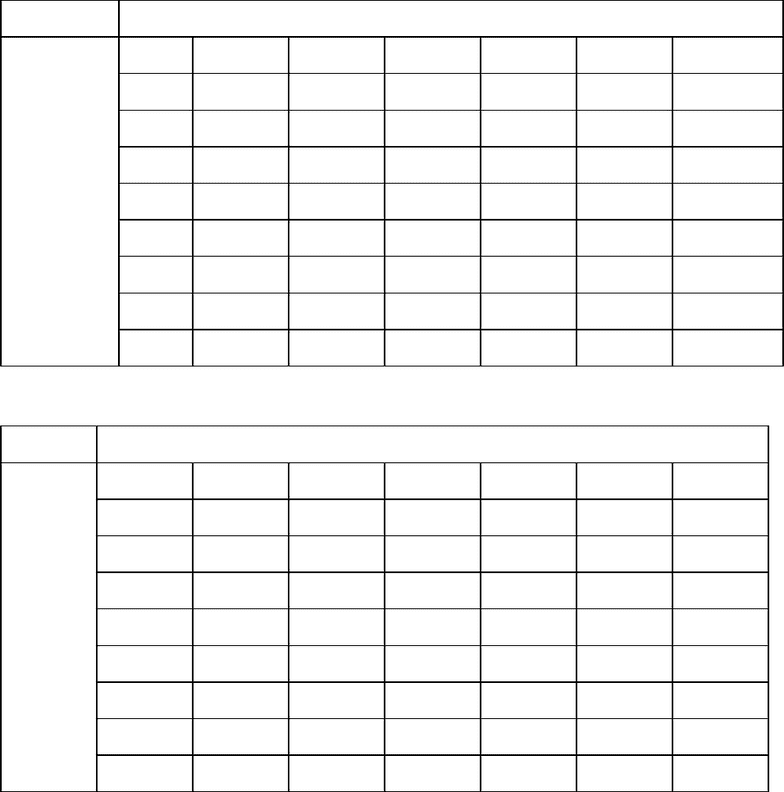
Table 1. General probability structure.
hair colour j=1,..,c Row sums
p11 p12 p1c p1.
p21 p22 p2c p2.
pij pi.
pr1 pr2 prc pr.
eye colour
i=1,..,r
Col sums
p.1 p.2 p.j p.c 1
Table 2. Independent Probability Structure
hair colour j=1,..,c Row sums
p1. p.1 p1.p.2 p1.p.c p1.
p2. p.1 p2.p.2 p2.p.c p2.
pi.p.j pi.
pr.p.1 pr.p.2 pr.p.c pr.
eye
colour
i=1,..,r
Col sums
p.1 p.2 p.j p.c 1
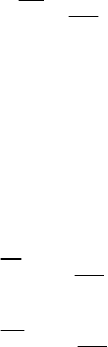
The Theoretical Implication of Independence.
Back in chapter 2 we observed that, if two events A and B were independent, their joint
probability was equal to the product of their marginal probabilities so that P(Aî…€B) = P(A)P(B)
under independence. If this is true for hair colour and eye colour then P(i’th eye colour and j’th
hair colour) = pij = P(i’th eye colour regardless of hair colour) x P( j’th hair colour regardless of
eye colour) = pi. p.j would have to hold for all i and j. This would change the configuration of the
probabilities in Table 1, Table 2 presents how they would look.
The sample structure.
Suppose we have a random sample of N individuals. Each one of the individuals in the sample
will have one and only one of the hair colour - eye colour category combinations. The number of
people in the sample with the ijth eye-hair colour combination (Oij) divided by the total number
of people in the sample (N) can be thought of as an estimate of the true probability that a
randomly selected person from the population has that particular hair colour-eye colour
combination so that the estimate may be written:
ij
ij
O
pN
î€
Similarly the sum over all possible eye colours (hair colours) of the number of people with the
i’th hair colour (j’th eye colour) divided by the total number of people in the sample can be
thought of as an estimate of the true probability that a randomly selected person from the
population has the i’th particular hair colour (j’th particular eye colour) so that:
1
1
rij
i
i
cij
j
j
O
pN
O
pN
î€
î€
î€
î€


These estimates obey adding up rules in the same way that the true probabilities obey such rules
namely: