Statistical Sciences 2035 Study Guide - Quiz Guide: Saber Of London, Principal Component Analysis, Sudoku
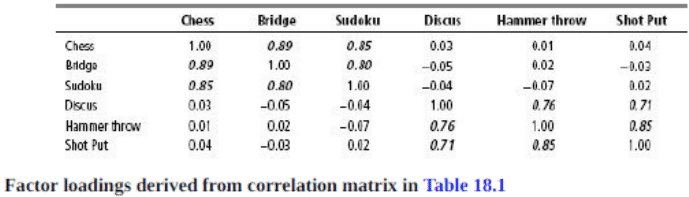
PRINCIPAL COMPONENT ANALYSIS
As there are an infinite number of equally accurate factor solutions for a given set of
correlations, researchers require a tool that chooses the most appropriate set. The
mathematically simplest is the Principal Component Analysis (PCA) that derives a
relatively small number of components that can account for the variability found in a
relatively large number of measures.
PCA in EFA is mostly used for:
- Data reduction, when a researcher does not want to include all of the original
measures in analyses but still wants to work with the information that they contain.
- Identification of the nature of the constructs underlying responses in a specific
content area
- Determination of sets of items ‘hang together’ in a questionnaire
- Demonstration of the dimensionality of a measurement scale
- Generation of ‘factor scores’ representing values of the underlying constructs for use
in other analyses
Assumptions for performing a PCA:
- Large enough sample to yield reliable estimates of the correlations among the
variables, i.e. large ratio of N /variables usually at least 5:1, preferably 10:1.
- Statistical inference is improved if the variables are at least approximately normal.
- Presence of linear relationships among the pairs of variables.
- Absence of outliers among the cases.
- Interval data.
Basic concepts and principles of PCA
A factor analysis usually begins with a correlation matrix:
We can detect two
distinct clusters of
high correlations,
namely, chess, bridge
and sudoku, and
discus, hammer and
shot put.
Document Summary
As there are an infinite number of equally accurate factor solutions for a given set of correlations, researchers require a tool that chooses the most appropriate set. The mathematically simplest is the principal component analysis (pca) that derives a relatively small number of components that can account for the variability found in a relatively large number of measures. Data reduction, when a researcher does not want to include all of the original measures in analyses but still wants to work with the information that they contain. Identification of the nature of the constructs underlying responses in a specific content area. Determination of sets of items (cid:494)hang together(cid:495) in a questionnaire. Generation of (cid:494)factor scores(cid:495) representing values of the underlying constructs for use. Demonstration of the dimensionality of a measurement scale in other analyses. Large enough sample to yield reliable estimates of the correlations among the variables, i. e. large ratio of n /variables usually at least 5:1, preferably 10:1.